Analytics Applications Every Marketer Should Know
Last Updated August 13, 2019
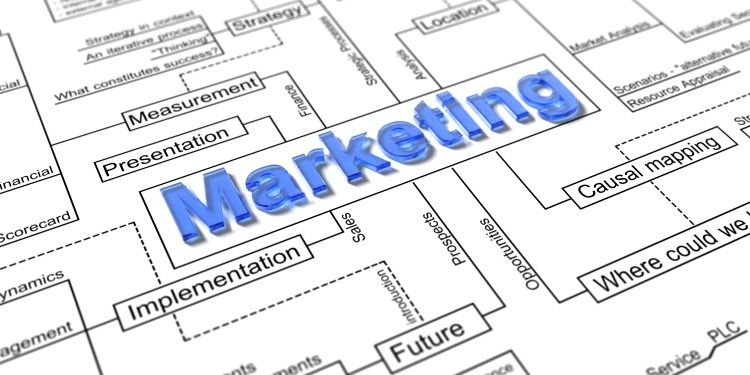
Analytics is a key component of any marketing strategy, integral to monitoring, measuring and projecting success of marketing campaigns. By obtaining a high-level view of data insights and applying the four types of data analytics—descriptive, diagnostic, predictive and prescriptive—marketers can enhance marketing campaigns and sales analysis in a variety of ways, while gaining a clearer picture of how their efforts impact the larger business organization.
Gaining Insights from Past Results
Marketing professionals can analyze to understand past results and the current market landscape with descriptive and diagnostic analytics.
Descriptive analytics helps marketers better understand who their customers are, how they behave and if sales results accurately reflect that by looking at historical data. This can include analyzing:
- Marketing investment
- Assigned dollar value to pipeline contributions
- Return on investment by channel
- The number of prospective customers who entered the sales funnel
- Customer segmentation to create audience personas
Marketers can then take these descriptive reports one step further with diagnostic analytics to understand why an event happened. For example, did the marketing department invest more in a channel that saw a very low return on investment? Uncovering this type of descriptive data can lead into diagnostic analytics to better understand why a certain result occurred. Marketers can review event action data to isolate an issue (i.e. a website glitch that affected sales) or an outside trend, such as the arrival of a new competitor in the market.
Diagnostic analytics can also establish cause and effect relationships within customer segmentation. For example, audience personas may include working, full-time students who are also parents, and working students who are not parents. Looking at customer data based on this segmentation could reveal the student persona with young children responded at very high rates to a 15% savings offer, while those students who are not parents preferred an offer of discounted expedited shipping.
Anticipating Trends
Anticipating market trends, sales dips and seasonal anomalies are important for marketers to create campaigns that drive sales and set trends. By applying predictive analytics, which is rooted in probability, marketers gain insight to what could happen based on past trends. Unlike diagnostic and descriptive analytics, which are rooted in the past, predictive analytics describes a potential future event.
For marketers, predictive analytics can help to estimate a social media score (also called sentiment analysis), forecast how many sales leads will be converted to customers or establish the likelihood a customer will cancel a product service.
Building from the historical information using techniques such as quantitative analysis, predictive modeling, artificial intelligence and machine learning algorithms, the predictive algorithm estimates a trend line and predicts a future outcome. Predictive analytics requires robust, accurate data set to create an accurate algorithm, as well as accurate data to train and develop the algorithm itself. In refining an algorithm, marketers must consider both macro and micro events to cultivate more accurate results.
For example, an initial time series analysis may predict a spike in website traffic in early-to-mid January, with a segmentation analysis showing working students drive January sales as they begin their spring semester. Based on this data, a campaign targeting this customer segment should go live separate from post-holiday sales campaigns. However, a review of additional historic data may remind the marketer that the prior year saw an unusual number of severe storms in January, which kept people at home. In that case, expanding the analysis to review additional years of January sales data or comparing to purchasing behaviors of the same customer segment in August before fall semester begins, can build the most accurate predictive model.
Accurately forecasting this supply and demand is where demand forecasting comes in. With demand forecasting, marketers can better understand the types of products and services customers are likely to seek, when they’re most likely to buy and adapt marketing strategies accordingly. And, thanks to those aforementioned robust data sets, marketers can drill down to specific regions or time frames to better understand who the customer is, what factors influence purchasing decisions and, therefore, when and what marketing campaigns should roll out. Demand forecasting can lay the foundation for other sales and marketing analytics through a better understanding of demand and the marketing support needed to influence it.
Proposing Future Campaigns
With a base of historical results and predictions toward probable trends or customer behaviors, it’s time to take new actions. Prescriptive analytics offers a highly refined level of insight that builds upon description and prediction to prescribe the most beneficial actions by making a set of recommendations, rather than just describing or correlating events. For marketers, this can mean pointing to the best channels for customer engagement or recommending relevant product upsells.
Online shoppers will recognize prescriptive analytics in the “suggested items.” These recommendation engines couple personal, descriptive data with predictive analytics to make personalized recommendations. By analyzing customer purchase data based on audience segmentation, marketers can learn how to best influence different segments of their customer base and refine their efforts with greater levels of personalized marketing that targets exact customers. In developing and refining prescriptive analytics algorithms, the value of the algorithm can be verified by the number of people who click on the product recommendation, sale offer or engage with the content.
For example, companies using marketing automation software can tie digital behavior data back to their customer relationship management (CRM) system, allowing for the tracking of customer engagement with email or mobile messaging campaigns, and apply appropriate data analytics to tailor future messages, content or product offers to individual customers. A targeted email campaign to working students with children may incorporate back-to-school savings on products for both the student and their children based on previous products purchased, related products and what’s trending for the season, while also incorporating personalized value-added content on effective study tools or ideas for balancing work, study and family time.
Constantly evolving technologies and data analytics applications will continue to enhance marketing and sales decisions and the possibilities for campaign creativity, changing the way companies market to consumers. Bolster your analytics skills and knowledge to gain that competitive marketing advantage.